Big Data Analytics
Quickly Access and Analyze Big Data with Infobright
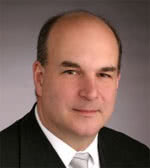
Data volume is growing exponentially, with many businesses struggling to keep pace with how to store and analyze the growing amount of critical business information. Infobright provides a scalable analytic database engine so that businesses can quickly and easily analyze machine-generated data without needing help from the IT department. President and CEO Don DeLoach shares why Infobright’s “cult-like focus” on machine-generated data can help organizations gain a comprehensive understanding of their data to optimize their networks and improve performance.
For more information about Infobright, visit their website and read our product review.
WEBSITE: www.infobright.com
FOUNDED: 2005
LOCATION: Toronto, Canada
SOLUTIONS: Infobright Enterprise Edition
How was Infobright founded? What was the inspiration to establish it?
Infobright was founded in 2005 by four mathematicians, three in Warsaw and one in Toronto. They created Infobright originally as a consulting firm specializing in data analytics. They recognized that the work that they were doing could be productized and very shortly thereafter they built what they called the Bright House Engine, which is basically the Infobright product, and attracted venture funding and got off to a traditional start as a start-up. In 2008 the company made the decision to really go into the market with what was now a new product.
Infobright’s market is a subset of Big Data Analytics. Can you tell us more about the type of market Infobright serves?
We did some loss analysis, we talked to customers, and we looked really hard at the product to determine the emerging world of machine generated data. If you look at the overall umbrella of Big Data, you looked at what the two biggest contributors were to Big Data, that being unstructured data and machine generated data. The table structures and the overall data structures associated with machine generated data are just really well suited for what we do. So if you happen to be storing call data records or web logs, or network security events—all of this is extremely well suited to be leveraged using the Infobright architecture.
More and more we focused really strictly on a subset of Big Data, that being machine generated data. Our customers are solution providers in the advertising technologies space, online analytics, mobile analytics, the manipulation of sensor data, online gaming, things like that. It works out really well, and the main characteristics are exceptionally low cost of ownership, very aggressive disc compression and an extremely good query performance, especially if there’s a need for doing ad hoc work. We’ve been able to keep that focus and leverage it in a very exciting way.
How would you characterize Infobright’s mission?
I would like for Infobright to be viewed as an innovative leader or one of the premier providers of very specific solutions for storing and analyzing machine generated data. We have an almost cult-like focus on machine generated data.
Who are some of your competitors and what do you do differently?
I guess the main competitors we see out there will be companies like Sybase IQ and Vertica. For the things that we do differently, let me cast a broader net. Sybase IQ or Vertica or other columnar stores are fundamentally analytic databases. There are very clever solutions out there. When I look at a Sybase IQ or a Vertica, I don’t think they’re necessarily the right answer in all issues—in fact, quite the opposite. But it’s a different architecture. What they have done at one level is to employ a similar strategy to ours in terms of using an inverted file structure where you’re storing data in columns, which tends to service analytic needs very well. They do that and we do that, and there are definitely some similarities there.
The difference is when the use case is machine generated data or what’s less important is the origins and what’s more important is the structure. But when the structure is conducive to our architecture we’re able to establish the architecture and maintain it without the need for database administrators, establishing and maintaining indexes, continually tuning the database, having to anticipate the types of queries that need to be asked so that we have significant advantage when ad hoc queries begin. And so we are different than them in so much as if the use case is storing and analyzing sheet generated data, we can deliver a more aggressive cost ownership and an easier to maintain environment than what you would get with one of the alternatives.
Now the flip side to this is that the people we compete with are generally able to address a broader set of use cases—what I would characterize as more general data warehousing. So if you look at the customer base for Sybase IQ or Vertica, you tend to end up in many cases with a more general data warehousing environment that for example, might maintain hundreds of tables and utilize the data across a complex snowflake schema—and that really isn’t well suited for our architecture. Not only do we not focus on that, we explicitly will not engage in opportunities with that as a prerequisite because it’s just not the right use for our architecture.
Think of it this way: if analytics interfaces were a transportation modality, if they were cars of some sort, a general purpose car might be a four door sedan that is suitable for a variety of different use cases. But, if what I’m trying to do is transport a family of seven, then a general purpose sedan isn’t going to do it; you might need something like a Suburban. Conversely, if what I’m trying to do is get through traffic in New York or London at the height of traffic and I need to get into narrow streets and weave through traffic then a scooter or a motorcycle is going to be a much more acceptable alternative. That doesn’t make a motorcycle bad; it doesn’t make a Suburban bad; it just makes it more suitable for a specific use case.
Let’s take the case of a motorcycle. A motorcycle will cost less; it has limitations relative to a four door sedan or Suburban; it’s only going to transport one person; and it can’t carry a lot of cargo. But again, for the specific use case, even though it costs less, and it’s likely to be far easier to maintain, it is a more desirable solution. And, that is indeed the case with most of the use cases that we work with where storing and analyzing machine generated data is what our users are trying to address. In that regard we’re able to provide them with the ability to do that by spending far less money yet still get highly desirable results.
What challenges does machine generated data pose to businesses and how does Infobright alleviate those challenges?
The challenge would be that the volume of data is growing exponentially. There’s been an increase in the number of users of mobile devices and the number of instances where sensor data is deployed. So for example, it used to be that the type of sensors that would be in a phone would be fairly limited. Now there are sensors that can do barometric readings, which will show up in somebody’s smartphone, which makes everybody aware of you. So the increases in the amount and the sophistication of devices that auto generate data is growing at such a high level that it’s creating an enormous load on the systems that store and analyze this data.
For example, if the data is stored in Oracle, or if the data is stored in MySQL or on a server, it tends to be manageable up to a certain point after which the queries start to run very long, the disc space starts to grow very, very large, especially if performance is an issue where in a traditional database like that you have to keep indexing the database in order to maintain your previous performance.
So really, Infobright allows customers to get beyond those challenges and take advantage of the opportunity they have for getting a much more comprehensive understanding of what they’re dealing with by being able to for example say, “Hey, I happen to be an extremely large mobile carrier and I’m trying to optimize my networks. But, it would be highly desirable if I could look at seven days order call record instead of three days, and it would be great if my analysts could get the results back from their queries in fifteen seconds as opposed to thirty minutes.” And those are the types of almost game changing opportunities that can be leveraged with the right technology.
Big Data right now is a very hot topic, what do you think has facilitated this rise in Big Data? Where do you see it headed and where do you see Infobright fitting into that landscape?
There are two main drivers. One is the enormous increase in the number and in the amount of changes in data, and the other is the increase in the amount of unstructured data. That includes the digitization of photographs, video, and documents and the facilties that allow for the storage and socialization of these documents up the point where companies and organizations have so much more information to contend with and need to be able to analyze this information across a variety of structures.
So in days of old where you had an IBM mainframe computer and everything was basically in a highly structured environment, the people’s knowledge of and thought process about how they dealt with the organizational information was pretty much contained to what was going to be within those ITM files or BPM files or whatever. In today’s world, it’s everything. It’s images, it’s videos, it’s massive IT logs, it’s any combination of data, up to and including traditional records that are generated in traditional role-based databases as well. But, that’s becoming a smaller and smaller percentage of the overall ecosystem of information that we’re going to face and have to deal with.
In order to comprehend and leverage the power of that information, I think people are beginning to recognize that there are specialized tools that can be used to very cost effectively store and comprehend what’s within that data. So the challenges are really understanding that there’s no silver bullet and you want to use the right tool for the right environment.
For example, if I’m storing loads and loads of pictures, I might want to use NoSQL document store database like MongoDB for example. If I’m storing lots of machine generated data, I might want to use Infobright. In fact, I also might want to understand how these various technologies co-exist.
I think that there’s many technologies out there that are ideally suited for pieces of the overall Big Data equation, and the challenge is to be able to have the various component technologies working together to solve the right business problem. That doesn’t say that there’s any one right answer other than the right answer for a specific business challenge.
Where do you see the Big Data segment headed in five years? What do you think will be the next big trend within this segment?
I’m going to sound very biased when I say this, and perhaps I am, but one of the key characteristics of where I see the Big Data market going in the next five years is that machine generated data will play a bigger and bigger part. I think that will manifest itself in the most obvious way—and in essence there is a sea change, this will be it—and that we’re going to begin to see a huge shift in communications. If you look at the work of the WC3 or the Tim Berners-Lee Semantic Web Initiative, it’s all about creating an environment that enables a whole new set of capabilities that did not exist before. So certainly that is one of the aspects of the next big wave.
The other thing is I think that you’ll see tighter and tighter integrations so the abstraction of the technology will get greater and greater and I’m sure you will see a preponderance of appliances. If you look at the world of database appliances today, they are most assuredly general purpose in nature. I think that as the world evolves, you’re going to see much more specialized capabilities that are purpose built, much like what we’ve seen in the way of security networking where you have the B&F appliances or things like that.
I think that there’s a lot of sophistication and I think that the sophistication at the technology level will be abstracted to where there’s a greater ease of use for any users to utilize this technology. I think that the real sea change will come in terms of machine to machine communications and again, underneath that all is a massive growth of machine generated data.
Other than Infobright, who do you think are the most interesting people or companies in your market segment right now and why?
There’s some incredible developments in terms of what’s going on with memory technology, so I think that that will affect how databases are architected in the future.
If you look at what people are doing with the innovation in sensor technology, there’s all kinds of coordination in technology. So, take the idea that you’ve got a Bluetooth phone. It’s been around for a while and is no great change. But what about the idea that you have sensors that you’re either imbedding inside your body or wearing on your body that are taking measurements of vital statistics and that understand where certain thresholds exist; and when you trip a threshold it activates a link to your cell phone via Bluetooth that’s been phoned into a central repository or a central monitoring environment that alerts a health organization that one of their patients has just tripped an alert? Or, ADT could monitor a house for burglary; only now it’s sophistication and technology combined with advance data communication that form this link.
So stuff like that I think is awesome. And I think it’s going to be way more widespread than anybody thinks and it’s going to happen way sooner than most people think.
What is next of Infobright?
We will not deviate one iota from our religious focus on machine generated data, but we will continue to introduce the ability to deal with greater and greater volumes of data, and utilize innovative and unique techniques for how that data is interrogated all in the name of better utilizing machine generated data.
Want to read more Business-Software.com exclusive interviews with CEOs and company founders? Head over to the Behind the Software Q&A section of the blog to browse the complete Behind the Software collection.